Mutf_In: Nipp_Indi_Flex_Fpecv2
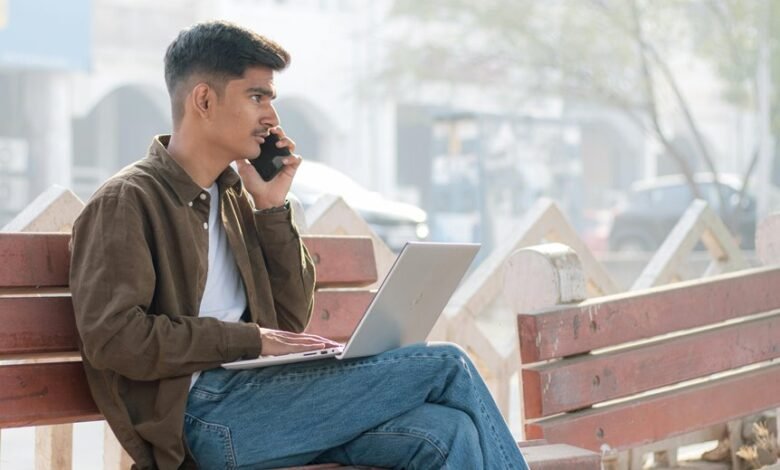
Mutf_In: Nipp_Indi_Flex_Fpecv2 represents a significant advancement in data structures, focusing on storage efficiency and retrieval speed. Its modular design allows for greater adaptability in various fields, such as healthcare and finance. By enhancing predictive modeling, it addresses limitations found in traditional approaches. However, the implications of this innovation extend beyond technical efficiency, raising important questions about ethical data usage and its impact on decision-making processes. What challenges might arise as these systems become more integrated?
Key Components of Mutf_In: Nipp_Indi_Flex_Fpecv2
The Mutf_In: Nipp_Indi_Flex_Fpecv2 is characterized by several key components that define its structure and functionality.
This innovative data structure optimizes storage efficiency and retrieval speed, ensuring robust performance metrics.
Its modular design allows for adaptability, promoting scalability and enhancing user experience.
Applications Across Various Domains
Applications of Mutf_In: Nipp_Indi_Flex_Fpecv2 span a diverse range of fields, showcasing its versatility and effectiveness in various contexts.
In healthcare analytics, it enhances patient outcomes through improved data interpretation.
Meanwhile, in financial forecasting, it aids organizations in making informed decisions based on predictive insights.
This adaptability across domains underscores its significance in driving innovation and efficiency.
Enhancing Predictive Modeling Efficiency
While many predictive modeling techniques struggle with large datasets, Mutf_In: Nipp_Indi_Flex_Fpecv2 offers an efficient solution by streamlining data processing and analysis.
Future Implications for Data-Driven Decision Making
As organizations increasingly rely on data-driven approaches, the implications for decision-making processes are profound.
Future strategies must prioritize data ethics and algorithm transparency to foster trust and accountability. By ensuring ethical practices, organizations can mitigate biases and enhance stakeholder confidence.
Ultimately, embracing these principles will empower decision-makers, allowing them to harness data effectively while respecting individual rights and societal norms.
Conclusion
In a world increasingly driven by data, one might expect that efficiency and transparency would reign supreme. Ironically, Mutf_In: Nipp_Indi_Flex_Fpecv2 emerges as a beacon of hope amidst the chaos of traditional data structures, promising streamlined processing and ethical practices. As organizations navigate the complexities of predictive modeling, they may find themselves pondering how such an advanced solution could have been overlooked for so long. Ultimately, the future of data-driven decision-making may hinge on embracing this innovative architecture.